The MScAI project
In numbers

• Sustainable energy
• Transportation / Mobility
• Healthcare / Biomedical
• Industry of the future
• AI for Finance
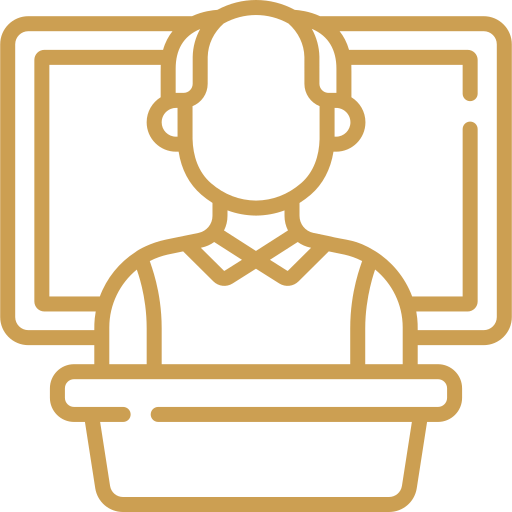

and 150 doctoral students engaged in this scientific field at CentraleSupélec

in Data Science and Artificial Intelligence at CentraleSupélec
Supervising team
The MSc AI team at CentraleSupélec is there to supervise and monitor students and ensure we meet your expectations. Delivering a program of excellence with enthusiasm, the team will support you as you develop AI skills that are adapted to the realities of society without ever losing sight of core concerns.
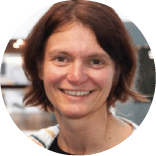
Céline Hudelot is Professor in Computer Science at CentraleSupélec. She heads the MICS laboratory (Mathematics and IT for Complex Systems). She is interested in both data-driven and knowledge-driven Artificial Intelligence for the semantic interpretation of non-structured data, with a particular interest in explainable AI (XAI).
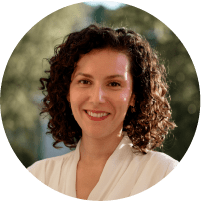
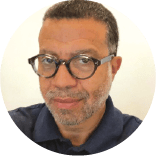
Dave Jacob brings his expertise in communication and development to the program. He has extensive international experience which is an asset when it comes to managing projects. He is a perfect fit with the objectives of the MSc AI, given the high stakes and the importance of ethics.
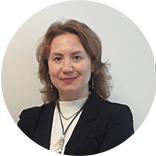
Teaching team
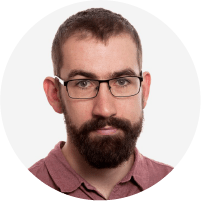
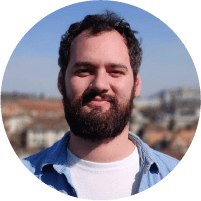
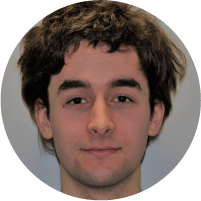
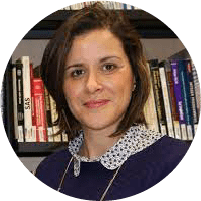
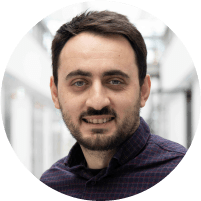
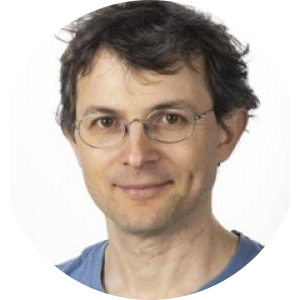
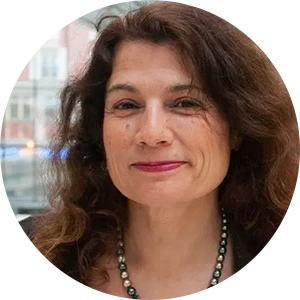
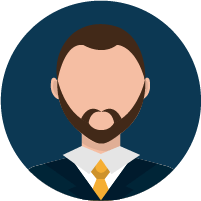
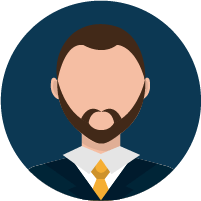
Perspectives
Artificial intelligence has become increasingly prevalent in our lives and is a definite game changer for society. This program aims to provide students with the foundations and most advanced techniques in the field, enabling them to become technical leaders of this transformation. Our program offers a unique curriculum, tackling the field with model / symbolic-driven and data-driven artificial intelligence methods, assessing their applications to key societal domains such as sustainable development, new mobilities, networks, Industry 4.0, finance and health / wellbeing.
This unique program, offering an end-to-end approach from theory to practice, is delivered entirely in English by outstanding academics and professionals, and offers an excellent curriculum to those preparing for a future as Artificial Intelligence architects seeking exceptional career perspectives in the hottest discipline of the 21st century.
As a French person, I was well aware of the reputation of CentraleSupélec. It is one of the best schools in France. Furthermore, the description of the course interested me a lot: Most schools that have AI programs are focused on Machine and Deep Learning but AI is not only about that. There are plenty of issues that exist in AI that Deep Learning would not solve. I would describe the MSc AI of CentraleSupélec as:
- Demanding: the program is short and intense
- Gobal: we study a lot of different fields in depth
- Balanced: we did both research opportunities and application.
Louis de Vitry,
Co-founder of Kanop